ECE Seminar Series – Jan 20 (Fri) @ 2:00pm: "Quantum-inspired Nonlocal Monte Carlo for Optimization and Sampling,” Dr. Masoud Mohseni, Google Quantum AI
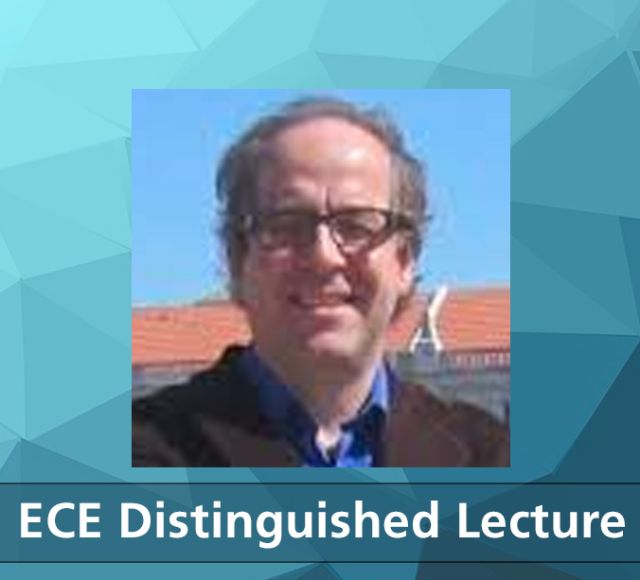
Come at 1:30p for Cookies, Coffee and Conversation!
DISTINGUISHED LECTURE at the ECE SEMINAR SERIES
Abstract
In this talk, I will present a new class of physics-inspired algorithms for sampling and discuss the possibility of building hybrid quantum-classical platforms for optimization and machine learning. In particular, I will introduce a quantum-inspired family of nonlocal Monte Carlo algorithms by developing an adaptive gradient-free strategy that can efficiently infer key geometrical features of the cost function in an instance-wise fashion. That information can be employed on-the-fly to construct nonequilibrium thermal fluctuations for collective moves at the various length scales, circumventing costly exploration versus exploitation trade-offs. I will also discuss the possibility of employing hardware accelerators to improve the performance of such algorithms.
Bio
Dr. Masoud Mohseni is a Senior Research Scientist at Google Quantum Artificial Intelligence Laboratory. He was formerly a research scientist and a principal investigator at the Research Lab of Electronics at MIT, where he was also a scientific consultant at BBN Technologies on unconventional computing. His current research addresses some of the hard problems at the interface of artificial intelligence, quantum computing, and statistical physics. He leads the development of quantum-inspired heuristic algorithms at Google. He led the development and management of the open-source software platform known as "TensorFlow Quantum," which was launched in 2020. He is also the tech lead for developing "IsingFlow," for physics-inspired optimization/sampling, on Google's distributed computing platform known as Borg, which can be used as an inference engine for probabilistic machine learning and training energy-based models. Dr. Mohseni has also contributed to the development of seminal quantum machine learning algorithms, including Quantum PCA, Quantum SVM, and Quantum state/process tomography, which in certain cases could lead to a provable exponential speedup when the input data is inherently quantum. He is a co-inventor of more than 20 patents at Google. He is currently writing a graduate-level textbook on "Quantum Machine Learning from First Principles," which is expected to be published by Cambridge University Press in 2023.
Hosted by: ECE Seminar Series
Submitted by: Kerem Camsari <camsari@ece.ucsb>