Camsari – Randomness for Probabilistic Computing
Kerem Camsari, an ass't. prof. of ECE, and colleagues conceive a device usually associated with stable information storage can be modified to be extremely sensitive to thermal noise, offering a source of randomness for probabilistic computing
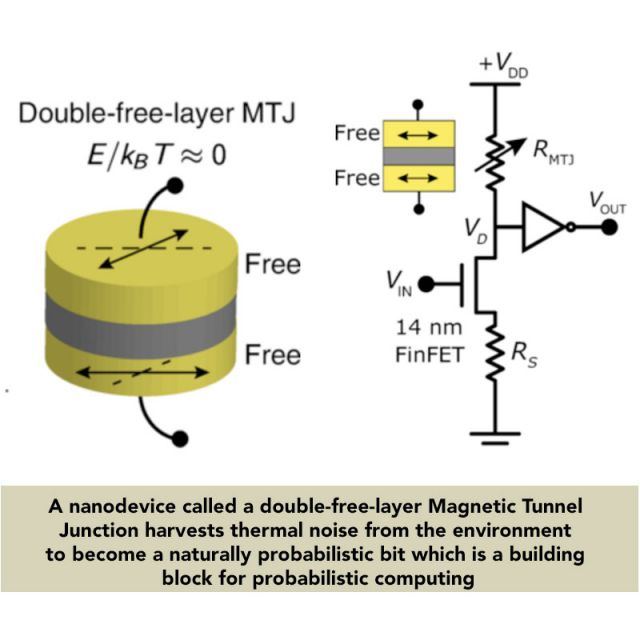
Maximizing Volatility in Magnetic Tunnel Junctions
Kerem Camsari at the University of California, Santa Barbara, and colleagues might have devised the worst possible computer-memory device. Their array of electronic structures—called magnetic tunnel junctions (MTJs)—have magnetic states that are so volatile that thermal noise causes the states to constantly change, making the MTJs incapable of retaining information [1]. But the device is far from useless. The researchers hope that its fluctuating electrical signals could be a valuable resource for probabilistic computing.
An MTJ consists of two nanomagnets separated by an insulating film. Conventionally, one of these nanomagnets is fixed while the other rotates freely. When the magnetizations align, electrons tunnel across the insulating barrier, producing a low-resistivity state. When the magnetizations are opposed, tunneling is suppressed, and the MTJ adopts a high-resistivity state.
MTJs have attracted interest because the stability of their states makes the devices promising platforms for information-storage applications. But the free nanomagnet in each device can be engineered to make the device sensitive to environmental noise, causing the states to spontaneously switch under a specific voltage. Such “stochastic” MTJs have been shown to be efficient sources of randomness.
Camsari and colleagues propose a variation on the conventional stochastic MTJ in which both nanomagnets freely rotate. Modeling their “double-free-layer” MTJ, they find that its states fluctuate faster than those of fixed-layer MTJs. Their device also retains its behavior over a wider range of voltages. The team says that the symmetrical design should make it easier to mass-produce their MTJs using existing techniques. Recently, they used a string of eight conventional stochastic MTJs to solve a simple factorization problem [2]. With their new double-free-layer design, they say they could harness thousands of MTJs to tackle more ambitious computations.