Miolane: NSF CAREER Award
ECE Assistant Professor Nina Miolane receives a National Science Foundation (NSF) Early CAREER award for her work in the field of computational geometric statistics
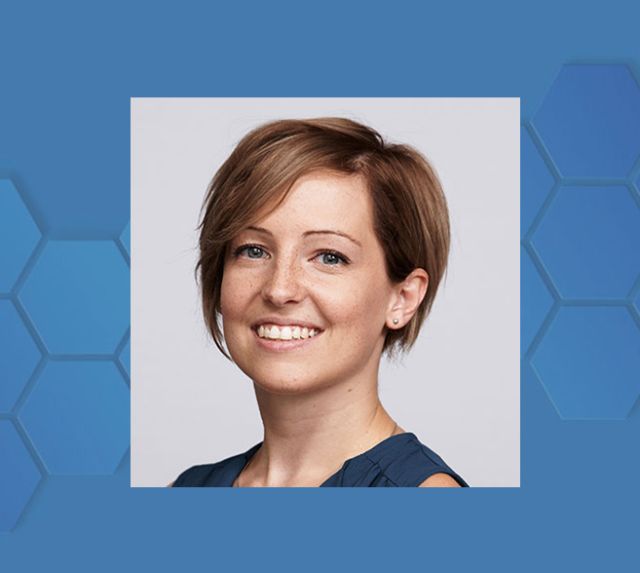
From the COE News article – "An NSF Early CAREER Award to Advance Geometric AI for Imaging Sciences"
In the past couple of decades, a new technology called 3D live imaging has expanded the medical community’s image-based research toolkit and made it possible to create videos of biological structures such as neurons and watch them interact in real-time at high-resolution. Because the technology generates enormous numbers of time-lapse images, it has benefitted from faster and more powerful computers. But, while live 3D imaging generates amazing videos, a challenge remains in terms of describing what the images actually show.
Live imaging of neurons is one example of the imaging revolution that is unfolding in the life sciences, with the sheer number of videos generated in these disciplines providing an incredible opportunity to ask novel scientific questions, including: What are the geometric signatures of neurodegenerative diseases, such as Alzheimer’s? and How do cognitive abilities arise from the geometry and topology of neuronal activity?
Answering such questions requires describing and quantifying geometric structures shown in images and videos. The field of Geometric AI has been created for this purpose, and the field of Geometric Statistics supports the results with a strong theory based in applied mathematics. The potential societal impact is immense. In a research setting, automated, large-scale geometric methods can accelerate the pace of groundbreaking discoveries. In a clinical setting, they can make the difference between a disease that we can identify quickly and one that remains a mystery for too long — determining whether or not intervention can occur in time to save lives.
Nina Miolane, an applied mathematician and an assistant professor in the UC Santa Barbara Electrical and Computer Engineering Department, has the field of computational geometric statistics to precisely quantify every nuance of geometries captured in live 3D images. Now she has received a five-year, nearly $500,000 National Science Foundation (NSF) Early CAREER award, the most prestigious NSF award for early-career faculty, to further develop those methods.
“I am honored to have this incredible opportunity to establish a long-term research program on a topic that I am passionate about, and thrilled to contribute to the mathematical and methodological developments of Geometric AI for imaging sciences,” Miolane says. “Above all, I am grateful to work with such fantastic graduate students and postdoctoral researchers. I would not have received this award without their commitment to excellence and their enthusiastic approach to research, outreach, and education. Congratulations to them!”
In awarding Miolane’s CAREER Award, the NSF wrote that Miolane will “evaluate and develop reliable shape-analysis methods that can harness the recent bio-imaging data explosion, advance our understanding of the fundamental rules of life, and enable breakthroughs in data-driven biomedicine.”
The proposal also included an education-and-outreach objective intended to engage diverse audiences in geometric AI through novel art-science performances for high-school students, pioneering courses on geometric deep learning, training of graduate students, and free community lectures for wider audiences.
Despite impressive advances in the field of shape analysis, computational and statistical hurdles prevent it from being deployed in life sciences. Research in geometric statistics began in the 1950s. Several decades ago, code was written for it, but it did not gain much traction, Miolane noted in a recent article in Convergence magazine. Her lab’s important advance, she says, has been “to implement the theory and create open-source code,” thereby “democratizing geometric statistics as a class of methods that can be used to automatically quantify motions and deformations from images.” As of August 2022, the open-source software she wrote for her method had been downloaded more than 120,000 times since being released in December 2020.
Miolane says that her long-term goal is to develop the field of geometric AI in the hope of accelerating the pace of groundbreaking discoveries in natural sciences and engineering. Her objective in her CAREER project, titled “Advancing Shape Learning for Biosciences,” is to evaluate and enhance methods that analyze the evolutions of shapes through time and predict their future.
COE News – "An NSF Early CAREER Award to Advance Geometric AI for Imaging Sciences"