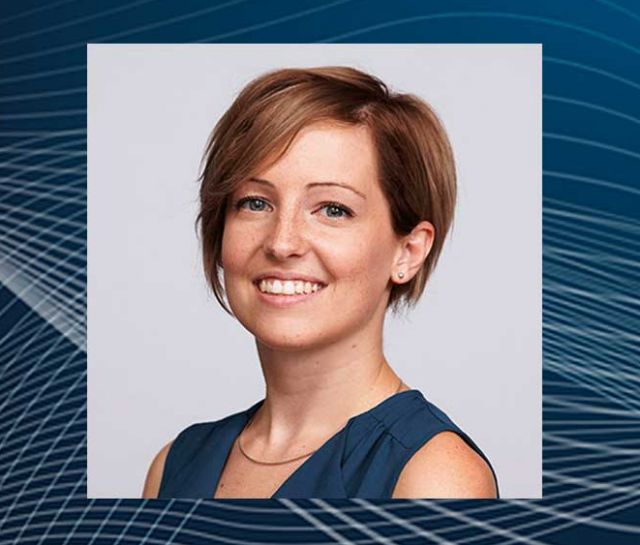
COE Q&A w/ New Faculty: Nina Miolane
UC Santa Barbara’s College of Engineering will welcome six new faculty throughout the 2021-22 academic year, including Nina Miolane, who is joining the Electrical and Computer Engineering (ECE) Department as an assistant professor.
Prior to arriving at UCSB, she was a postdoctoral researcher at Stanford University and a software engineer at Atmo, working on deep learning and dynamical systems methods in image processing, and at Caption Health, developing medical imaging algorithms for computational medicine. Miolane earned a bachelor’s and masters degree in mathematics and theoretical physics from Ecole Polytechnique in France, and a master’s degree in theoretical physics from Imperial College in the United Kingdom. She earned her PhD in geometric statistics for computational anatomy from the National Institute for Research in Computer Science and Automation (Inria) and Université Côte d’Azur (UCA) in France, in collaboration with Stanford.
Here's what she had to say:
Q: Please explain a bit about where you have worked or taught at prior to coming to UCSB?
A: Prior to coming to UCSB, I contributed to computational biology and medicine research in academia and industry. My PhD research investigated fundamental mathematics for the development of "computational anatomy", an emerging field modeling organ shapes, with applications to the study of neurodegenerative diseases. As a software engineer at Caption Health, I developed techniques for the diagnosis of cardiovascular diseases from echocardiographies. As a postdoc at Stanford, I extended the theoretical approach from my PhD to develop computational tools for brain and biological image processing. Most recently I worked as a software engineer at Atmo, where I investigated methods at the intersection of deep learning and dynamical systems in order to process giant imaging datasets. I am excited to pursue the work in biomedical imaging for computational biology and medicine at UCSB.
Besides these research activities, I taught the class “Statistical Methods for Engineering and the Physical Sciences“ at Stanford in 2018 and 2019. I have also been involved in outreach initiatives in San Francisco with the goal of exposing state-of-the-art research to a broader community beyond academia, and projects with French high schools to advocate for diversity in science.
Q: What is the focus of your research?
A: My research contributes to building theoretical foundations and developing practical tools to create computational representations of biological structures — organs, cells, molecules — from biomedical images. Modeling the healthy and pathological states of these structures is crucial for applications in biology and medicine, ranging from our understanding of cancers to the diagnosis of neurodegenerative diseases.
Specifically, I focus on quantifying biological shapes and their variations in biomedical imaging datasets -— using tools from geometry, statistics, machine learning, deep learning and computer vision. Such "shape descriptors" are then correlated with healthy or pathological conditions, thanks to collaborations with biologists and clinicians within academia or industry.
Q: How did you get interested in these specific fields of research?
A: My interest in computational medicine is motivated by the ideal of "democratized" medicine. Automatic diagnosis methods implementable in computers can theoretically be duplicated as many times as needed, disseminated across regions regardless of their economic status, and provide either a virtual assistant to local doctors, or a crucial initial screening in areas that have a shortage of medical specialists. I believe that computational medicine can help provide foundations for medical standards to all.
From a methodological perspective, I am also particularly excited about the tools required to perform this research. They couple state-of-the-art deep learning techniques with mathematics inspired from the developments of theoretical physics in differential geometry. The intersection of the two fields is fascinating.
Q: What are the real-world implications of your research?
A: By mining biomedical imaging datasets with innovative methods, we expect machines to detect subtle physiological patterns that might be invisible to the naked eye of a biologist or a clinician. We anticipate achieving new findings about biological processes, and hope to develop cutting-edge computer-assisted diagnosis methods. The goal of this research is to push forward the fields of biology and medicine and contribute to their transformation into statistically grounded and computationally enabled disciplines.
Q: You started an open-source project, Geomstats, a few years ago with collaborators around the world. What is Geomstats, and how has it led to a fruitful collaboration between mathematicians and engineers?
A: Geomstats is a Python library that contains computations and statistical learning algorithms for data spaces that have specific geometric properties. For example, Geomstats allows users to perform clustering or dimension reduction on datasets of shapes, such as shapes of cancer cells that have been exposed to different drugs.
The advances in biomedical imaging techniques have enabled us to access the 3D shapes of a variety of structures: organs, cells, proteins. Since biological shapes are related to physiological functions, biomedical statistical analyses are poised to incorporate more shape data. Yet, the mathematics involved with computing with shape spaces can rely on subtle concepts from differential geometry. Geomstats is a platform where: (i) on the one hand, mathematicians can contribute code involving differential geometry; and (ii) on the other hand, users (such as engineers or researchers from other fields) can use this code without having to delve into the mathematical concepts. It has led to many fruitful collaborations!
Q: How excited are you to join the Electrical and Computer Engineering Department at UCSB?
A: I am beyond excited to be joining the ECE department at UCSB! Everybody has been extremely welcoming from the job interview invitation to today. Since I started to work remotely, faculty members, administrative and technical-support teams have also been very responsive. I am grateful for the help they provided, and I cannot wait to meet and thank everyone in person. Besides, I am genuinely excited by the research going on in the department and I look forward to interesting scientific interactions and collaborations.
- Q: When did you move to the Santa Barbara area, and how are you adjusting to your new hometown?
- Q: Have you discovered a favorite beach or found a new favorite restaurant since you’ve moved to the Central Coast?
- Q: Tell us something interesting about yourself unrelated to work and research.
COE News – "Q&A with New CoE Faculty: Nina Miolane" (full article)